The sum of squared deviations, also known as the sum of squares, is a statistical measure used to determine the deviation of data points from the mean value. It is an important tool used in data analysis to understand the variability of the data and to draw conclusions based on the data.
To calculate the sum of squared deviations, we first need to calculate the sample mean. The sample mean is the average of all the data points in a sample. Once we have the sample mean, we can subtract it from each individual value in the sample. This gives us the deviation of each data point from the mean.
Next, we square each deviation to eliminate negative values. Squaring the deviations also emphasizes larger deviations from the mean, giving us a better understanding of the variability of the data. we add up all the squared deviations to get the sum of squares.
The sum of squares can be used to calculate several other statistical measures, such as the variance and standard deviation. The variance is the sum of squares divided by the number of data points minus one. The standard deviation is the square root of the variance.
A higher sum of squares indicates higher variability in the data, wile a lower result indicates low variability from the mean. This information is useful in determining the reliability of data and drawing conclusions based on the data.
The sum of squared deviations is an important statistical measure used in data analysis to determine the deviation of data points from the mean. It is calculated by subtracting the mean from each data point, squaring the deviations, and adding them up. The sum of squares is used to calculate other statistical measures such as the variance and standard deviation. Understanding the sum of squares is essential in data analysis to draw reliable conclusions based on the data.
How Do You Find The Sum Of Squared Deviations?
To find the sum of squared deviations, you need to follow a few simple steps. First, calculate the sample mean by adding up all of the values in the dataset and dividing by the total number of values. Next, subtract the mean from each individual vaue to determine the deviation from the mean for each value. Then, square each deviation to eliminate negative values and calculate the individual squared deviations. Finally, add up all of the squared deviations to find the sum of squared deviations. This calculation helps to determine the amount of variance or spread in a dataset from the mean value. By following these steps, you can easily calculate the sum of squared deviations in any dataset.
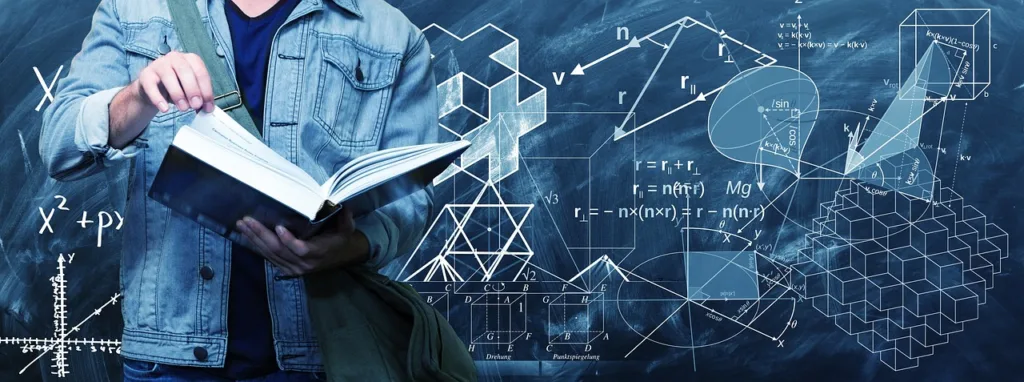
What Does Sum Of Squared Deviations Tell You?
The sum of squared deviations is a statistical measure that quantifies how far individual data points deviate from the mean value of a dataset. The result of the sum of squared deviations provides information about the variability of the data points with respect to their mean value. A higher sum of squares indicates that the data points are more widely spread out from the mean, indicating greater variability. On the other hand, a lower sum of squares suggests that the data points are closer to the mean, indicating lower variability. By calculating the sum of squares, we can evaluate the extent of variability in a dataset, whih is useful in various statistical analyses and decision-making processes. To calculate the sum of squares, we subtract each data point from the mean, square the differences, and sum up the squared values.
How To Do Sum Of Squared Deviations In Excel?
To do the sum of squared deviations in Excel, you can use the DEVSQ function. This function calculates the sum of squared deviations from the mean of a set of values. To use this function, you need to provide at least one argument, which is the range of cells that contains the data you want to analyze.
Here are the steps to use the DEVSQ function in Excel:
1. Select a cell where you want to display the result of the DEVSQ function.
2. Type the formula “=DEVSQ(” in the cell.
3. Select the range of cells that contains the data you want to analyze.
4. Close the bracket and press enter to calculate the sum of squared deviations.
If you want to calculate the sum of squared deviations for multiple sets of data, you can repeat the above steps for each set of data and add the results together.
It is important to note that the DEVSQ function assumes that the data is a sample of a larger population, not the entire population. If you have the entire population, you should use the VAR.P function instad of the DEVSQ function.
Using the DEVSQ function in Excel is a quick and easy way to calculate the sum of squared deviations from the mean of a set of values.
What Is Sum Of Deviations?
The sum of deviations is a statistical measure that quantifies the variation of a set of data from its mean value. It represents the total difference between each individual data point and the mean of the whole set. Mathematically, the sum of deviations is obtained by adding up the differences between each data point and the mean, where the deviations below the mean are negative and the deviations above the mean are positive. The sum of deviations is always zero, due to the property of the sample mean. However, it is a ueful measure to capture the magnitude of these deviations and to understand the dispersion of the data. By calculating the sum of deviations, we can determine the average distance between each data point and the mean, which is a key concept in statistics and data analysis.
Conclusion
The sum of squared deviations from the mean is a powerful statistical tool that measures the variability of a given dataset. By calculating the squared differences beteen each data point and the mean, we can get a clear picture of how dispersed the data is. A higher sum of squares indicates a wider range of values, while a lower result suggests that the data is more tightly clustered around the mean. The sum of squares is a crucial component in many statistical analyses, such as regression models, hypothesis testing, and ANOVA tests. By understanding how to calculate the sum of squared deviations, we can gain valuable insights into the underlying patterns and trends in our data, and make more informed decisions based on these findings.