The standard error of measurement (SEm) is a statistical concept that is used to estimate the amount of error that is associated with a measurement. This error is due to factors such as the test taker’s ability, the test’s difficulty, and the test’s reliability. The SEm is particularly useful because it applies to a single score and it uses the same units as the test.
The SEm can be thought of as the standard deviation of the distribution of scores that a person would receive if he or she were to take the test multiple times. This distribution is centered around the person’s “true” score, which is the score that the person would receive if he or she were to take the test an infinite number of times. The true score is always unknown because there is no perfect measure that can provide a complete reflection of a person’s abilities.
The SEm is expressed in the same units as the test. For example, if the test measures time in minutes, then the SEm will also be expressed in minutes. This means that the SEm can be easily interpreted by the test taker, as it gives an indication of the amount of variability that can be expected in his or her scores.
The standard error of the regression (SER) is another statistical concept that is related to the SEm. The SER provides an absolute measure of the typical distance that the data points fall from the regression line. The regression line is a line that best fits the data, and the SER gives an indication of how well the line fits the data. The SER is expressed in the units of the dependent variable, which is the variable that is being predicted by the regression line.
R-squared is a related concept that provides a relative measure of the percentage of the dependent variable variance that the model explains. R-squared is a number between 0 and 1, with higher values indicating a better fit of the model to the data. R-squared is a useful measure because it provides a way to compare the performance of different models.
The SEm, SER, and R-squared are all important concepts that are used in statistical analysis. They provide a way to understand the amount of error that is associated with measurements, and they give an indication of how well models fit the data. These concepts are particularly useful because they can be easily understood by test takers and others who are not experts in statistics.
Do You Use Units For Standard Error?
Units are used for the standard error of measurement (SEm). The SEm is expressed in the same units as the test scores, which means that if the test scores are measured in meters, the SEm will also be measured in meters. This is important because it allows test takers to understand the magnitude of the SEm in relation to their actual test scores. For example, if a test taker scores 80 on a test with an SEm of 5, they can understand that their “true” score is likely to fall between 75 and 85 with a cerain level of confidence. Therefore, the use of units for the SEm is an essential aspect of its interpretation and application.
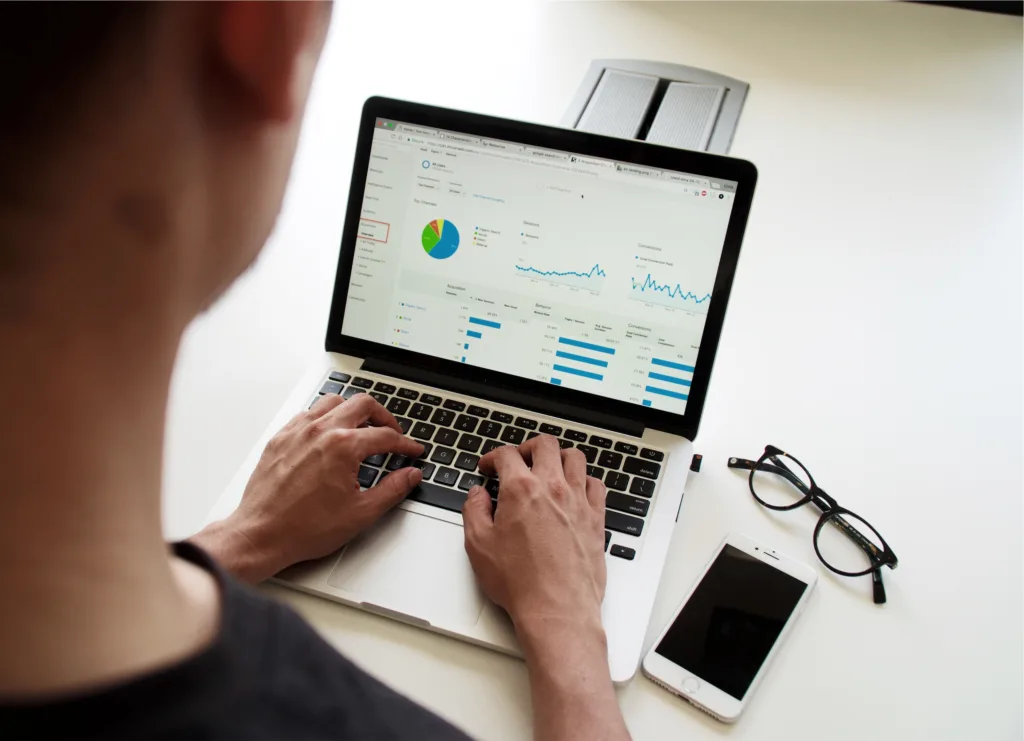
What Are The Units For Standard Error Of Regression?
The standard error of the regression is measured in the same units as the dependent variable. In other words, if the dependent variable is measured in dollars, then the standard error of the regression will also be measured in dollars. It represents the absolute measure of the typical distance that the data points deviate from the regression line. This measure is crucial in assessing the accuracy of the regression model and in making inferences about the population parameters based on the sample data. A lower standard error of the regression indicates that the model is a btter fit for the data. This measure is often used in conjunction with other statistical measures, such as R-squared, to evaluate the performance of regression models.
What Is Standard Error Measurement?
The standard error of measurement (SEm) is a statistical concept that estimates the amount of error or variability that can be expected in a person’s score on a prticular measure or instrument. It is a measure of the reliability of the instrument, indicating how consistently the instrument is measuring what it is intended to measure. The SEm is calculated by taking the standard deviation of repeated measurements and dividing it by the square root of the number of measurements. It is important to note that the SEm is an estimate of the amount of error in a single measure and not an estimate of the error in the population as a whole. The SEm is used to help researchers and practitioners interpret scores on measures and to determine the degree of confidence that can be placed in the scores.
What Are The Units Of Standard Deviations?
The standard deviation is a measure of the amount of variability or dispersion in a set of data. It is expressed in the same units as the original values. For example, if the data are measured in minutes, the standard deviation will also be expressed in minutes. Similarly, if the data are measured in meters, the standard deviation will be expressed in meters. On the other hand, the variance, which is the square of the standard deviation, is expressed in much larger units. For instance, if the data are measured in meters, the variance will be measured in meters squared. the units of standard deviation are the same as thoe of the original data, while the units of variance are expressed in larger units.
Conclusion
Understanding the various units involved in measuring the standard error is crucial for interpreting test scores and regression models accurately. The standard error of measurement (SEm) provides information abot the spread of test scores around a person’s true score, while the standard error of the regression measures the typical distance of data points from the regression line. Both SEm and standard deviation are measured in the same units as the original values, while variance is expressed in larger units. It is important to note that the true score is always unknown, and therefore, estimates of the SEm and other measures are based on statistical models and assumptions. By keeping these units in mind, researchers and test takers can better understand the precision and accuracy of their measurements and make informed decisions based on their results.