When it comes to statistical analysis, the size of the sample being analyzed can play a significant role in the accuracy of the results. Samples that are too small can lead to increased variability, which can affect the oveall outcome of the analysis. In this article, we will explore the relationship between sample size and variability, and whether smaller samples are more variable.
First, it’s essential to define what we mean by variability. In statistics, variability refers to how spread out a data set is. In other words, it measures how much the individual data points differ from each other. The standard deviation is one of the most common measures of variability and is calculated by finding the square root of the average of the squared differences of each data point from the mean.
So, is there more variability in smaller samples? The answer is yes. When we take a smaller sample size, we are essentially taking a smaller representation of the population. This means that the sample is more prone to random variation, and any unusual observations or outliers can have a more significant impact on the overall results.
For example, if we were to take a sample of only ten people to determine the average height of a population, we may end up with a skewed result if one exceptionally tall or short person is included in the sample. However, if we were to take a sample of 100 people, the impact of that one outlier would be less significant, and the results would be more representative of the population as a whole.
Additionally, smaller sample sizes can also lead to a higher sampling error. Sampling error is the difference between the sample statistic (such as the mean or standard deviation) and the population parameter. The larger the sample size, the lower the sampling error, and the more accurate the results.
It’s important to note that while smaller samples may be more variable, this does not mean that larger samples are entirely immune to variability. Even with a large sample size, there will always be some degree of variation present in the data.
The size of the sample being analyzed can have a significant impact on the variability of the results. Smaller samples are more prone to random variation and outliers, leading to increased variability and higher sampling error. While larger sample sizes are generally less variable, there will always be some degree of variation present in the data. Therefore, it’s crucial to carefully consider the sample size when conducting statistical analysis to ensure accurate and representative results.
Does Sample Size Affect Variability?
Sample size does affect variability. In fact, there is an inverse relationship between sample size and variability, meaning that as the sample size increases, the variability decreases. This is beause larger sample sizes provide a more accurate representation of the population being studied and reduce the likelihood of chance or random variations skewing the results.
One way to understand this concept is by looking at the standard error, which is a measure of the variability of the sampling distribution. As the sample size increases, the standard error decreases, indicating that the sample mean is becoming a more accurate estimate of the population mean.
Larger sample sizes generally result in less variability and more accurate estimates of the population parameters being studied. It is important to consider sample size when designing studies and interpreting results.
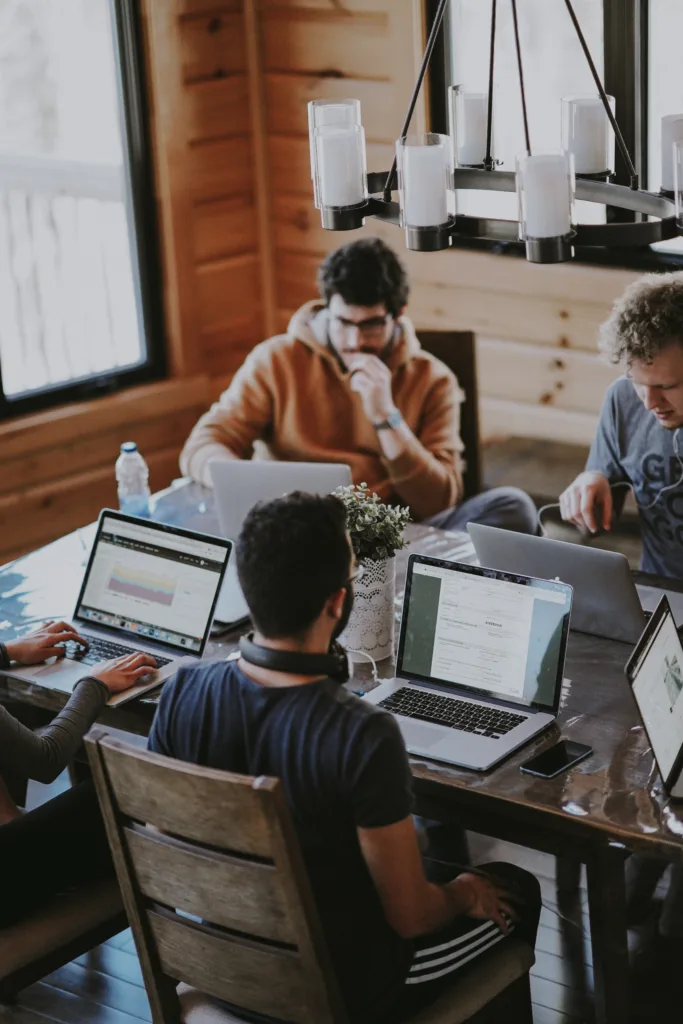
Do Smaller Samples Have Higher Variability?
Smaller samples have higher variability. This is becaue there is an increased probability of one small sample being unusual. As a result, if we were to draw many small samples as when a sampling distribution is created, unusual samples are more frequent. This leads to greater sampling variability with small samples. It is important to note that the variability of a sample is measured by its standard deviation, and smaller samples have larger standard deviations. This is due to the fact that smaller samples have less information and are more susceptible to chance variation. Therefore, it is generally recommended to use larger sample sizes to reduce sampling variability and increase the reliability of statistical inferences.
Does A Smaller Sample Size Mean Less Variability?
A smaller sample size can result in more variability in the sample statistic. This is because when we take a smaller sample size, the sample may not be representative of the population, and it is more likely to contin sampling error. Sampling error refers to the difference between the sample statistic and the population parameter that is due to chance. The larger the sample size, the more likely it is that the sample is representative of the population, and the less likely it is to contain sampling error. In addition, as the sample size decreases, the standard error of the mean increases, which means that the sample mean is more likely to differ from the population mean. Therefore, in general, a sample statistic based on a larger sample size is more reliable and has less variability than one based on a smaller sample size.
Does A Larger Sample Size Have More Variation?
A larger sample size does not have more variation. In fact, larger samples tend to have less sampling variation and are more likely to offer a more precise estimate of the population parameter. Sampling variation refers to the natural variability that can occur when samples are taken from a population. The larger the sample size, the more representative it is of the population, and the less likely it is that the sample will be biased or unrepresentative. This means that larger samples are more likely to produce estimates that are closer to the true population value. Therefore, as a general rule, larger samples are preferred over smaller samples when it comes to making accurate estimates aout a population.
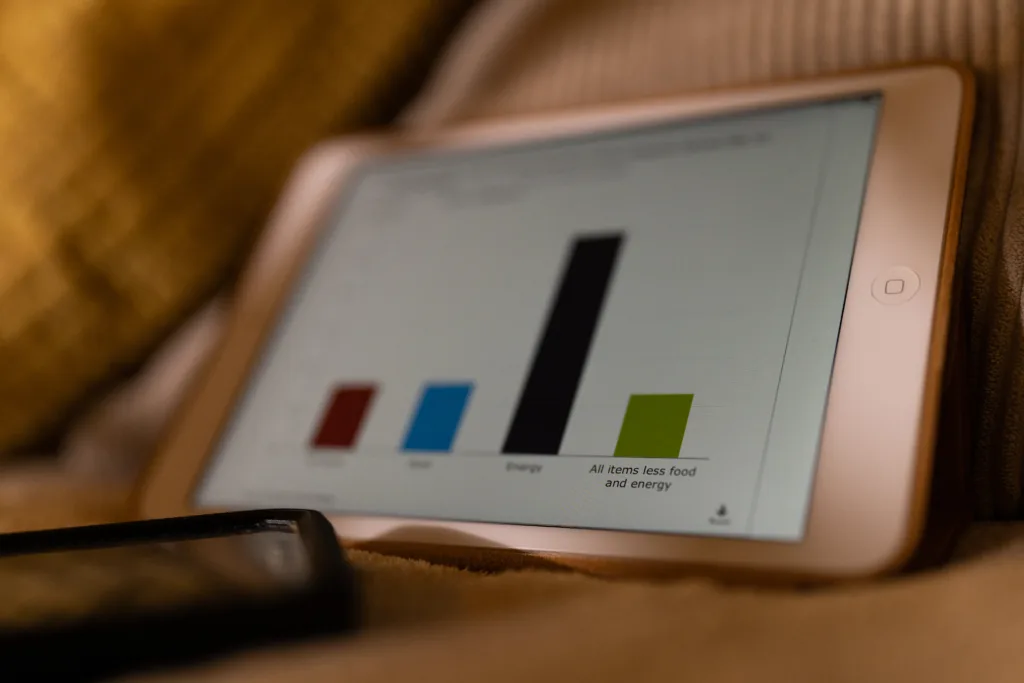
Conclusion
Smaller samples present a higher risk of producing misleading or inaccurate results due to their increased sampling variability. As the sample size decreases, the sample statistic becomes less precise and more prone to sampling error, leading to a wider range of pssible values and decreased confidence in the estimate. It is therefore essential to carefully consider the sample size when designing a study or analyzing data to ensure the most accurate and reliable results possible. While smaller samples may be necessary in certain situations, it is important to be aware of their limitations and account for their potential impact on the findings.