When it comes to studying the effects of an intervention on an outcome, it’s important to understand the difference between a covariate and a confounder. Both can affect the results of your study, but understanding how they are different will help you ensure that your findings are accurate.
A covariate is a continuous variable that is expected to vary with the outcome of the study. This means that any measurable quantity that has a statistical relationship with the dependent variable can be used as a covariate. This culd include age, gender, or socioeconomic status among others. By controlling for these variables you can minimize the potential sources of error in your results and make sure that only true relationships between variables are being detected.
On the other hand, a confounder is a variable that affects both the intervention and outcome variables in a study. It’s important to account for this when designing experiments because if not controlled for, it can mask or distort true relationships between other variables in your study. For example, if you’re looking at how effective an exercise program is at improving cardiovascular health, then factors like diet or lifestyle habits would need to be taken into account when analyzing data as they may be confounding factors in determining whether or not someone enjoys improved cardiovascular health from exercise.
It’s important to remember when designing experiments and analyzing data that both covariates and confounders could influence your results in some way or another. Accounting for them will help ensure that you get accurate results and avoid any bias caused by these confounding factors.
The Difference Between Covariate and Confounding Variable
The primary difference between a covariate and a confounding variable is that a covariate explains variability in the outcome, while a confounding variable introduces bias and obscures the true relationship between an intervention and an outcome. A covariate is usully an independent variable that is measured alongside the main independent variable(s) of interest, whereas a confounding variable is usually an extraneous or uncontrolled factor that may be associated with the outcome but is not part of the causal pathway. For example, in a study examining the relationship between smoking and cancer, age could be considered a covariate because it explains some of the variation in cancer risk among smokers; however, socioeconomic status could be considered a confounding variable because it could influence both smoking behavior and cancer risk independently.
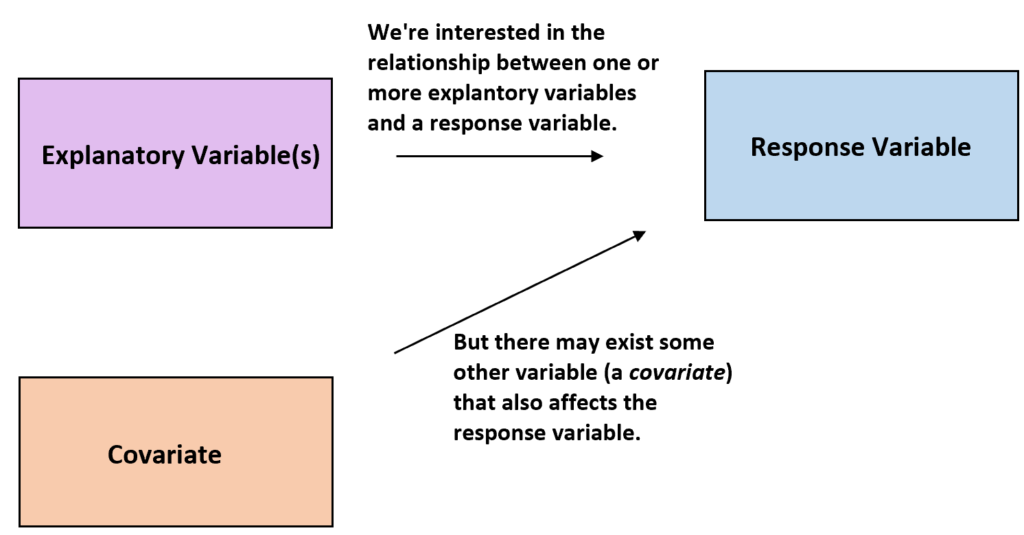
Are Covariates and Control Variables the Same?
No, a covariate is not the same as a control variable. A covariate is a type of control variable, but thre are other types of control variables as well. A covariate is an independent variable that may have an effect on the outcome of a study or experiment, but is not the primary focus of the research. It is used to help reduce variations in the results and make them more accurate. Control variables are used to ensure that any changes in the dependent variable are actually due to the independent variable and not something else. They can be used to control for both known and unknown factors that could affect the outcome of an experiment.
Determining If a Variable is a Covariate
To determine if a variable is a covariate, one should first identify the dependent variable of interest. After doing so, it is necessary to analyze the data to determine if there is any statistical relationship between the potential covariate and the dependent variable. This can be done by performing various tests to assess the correlation between the two variables, such as a Pearson correlation coefficient or a t-test. If there is evidence of significant correlation between the two variables, then it can be considered that the potential covariate is indeed a covariate. Additionally, it is important to conider whether or not any outliers might exist in the data that could affect this relationship and should be eliminated prior to testing for correlation. Once all of these criteria are satisfied, one can confidently say that a given variable is indeed a covariate.
The Role of Covariates in a Study
Covariates are an important tool used in many studies to examine the relationship between a response variable and one or more independent variables. A covariate is a continuous variable that is thought to have an influence on the outcome of a study. It can either be a predictor of the response variable or be affected by it. Covariates are often used when the researcher wishes to control for potential confounding variables, such as age, gender, ethnicity, etc., and can help to focus on the effect of one prticular factor while taking other factors into consideration. For example, if studying the effect of exercise on health outcomes, age could be included as a covariate to account for any differences in health status among different age groups. Additionally, including covariates can help to increase the power of statistical analyses and improve estimates of model parameters.
Can a Covariate Act as Both a Confounder and an Effect Modifier?
Yes, a covariate can be both a confounder and an effect modifier. A confounder occurs when the stratum-specific measures are similar to each other, but at least 10% different than the crude measure. In this case, the covariable is distorting the relationship between the exposure and the outcome. An effect modifier occurs when the stratum-specific measures are different from each other and the crude lies between them. In this case, there is a true difference in effect based on levels of the covariate.
What is a Covariate?
A covariate is a continuous, independent variable that researchers measure in an experimental design, usually alongside the primary factors of interest. Covariates are distinct from the primary factors in that they are uncontrollable and can’t be randomized — they are considered “nuisance” variables since they are external to the experiment’s design. Covariates can be useful for creating models which take into account additional information about the context of the experiment, allowing for more accurate predictions and analysis. For example, if a researcher is studying the effects of a particlar drug on an outcome, they may measure age as a covariate to better understand how age affects the outcome in addition to the effect of the drug.
Examples of Covariate Variables
Covariate variables are variables that can influence the relationship between the variable of interest and the outcome of a study. Common examples of covariates include age, sex, income, education level, health status, lifestyle factors (e.g., smoking status), and environmental factors (e.g., exposure to pollutants).
In epidemiological research, controlling for covariates is important in order to eliminate their potential confounding effect on the relationship between an exposure and an outcome of interest. By controlling for these variables, researchers can draw more meaningful conclusions about the association between the exposure and outcome.
In clinical trials, covariates are ofen used to identify subgroups that may respond differently to a given intervention or treatment. For example, if a drug is being tested for efficacy in treating diabetes, age may be a covariate used to identify whether older people respond differently than younger people.
In economics research, covariates often include socio-economic characteristics such as income level or education level which may influence an economic outcome such as purchasing behavior or labor force participation.
Overall, any variable that is thought to have an effect on the relationship between the variable of interest and an outcome can be considered a covariate.
What Is an Alternative Term for Covariate?
Explanatory variable is another term for covariate. A covariate is an independent variable that is used to explain or predict a dependent variable in a statistical model. It typically represents a factor that could have an effect on the outcome of an experiment or study, and that can be measured and monitored during the experiment or study in order to understand its impact on the results.
Is Covariate Always a Continuous Variable?
No, covariates are not always continuous. Depending on the study and the context, covariates can be either continuous or categorical. For example, in a study looking at the effect of gender on math ability, gender would be considered a categorical covariate. In contrast, age could be considered a continuous covariate in this same study. It is important to consider both types of covariates when designing and analyzing research studies.
When to Utilize a Covariate
Covariates should be used whenever there is potential for initial group differences that could affect the outcome of a study. This includes experiments that involve comparing two or more groups, such as in an ANCOVA study. Covariates can help to control for thse initial group differences and ensure that any observed effects are due to the independent variable being studied, rather than confounding factors. Additionally, covariates can be used to adjust for any underlying trends or correlations between variables, allowing for more accurate results. In summary, covariates should be used when there is a possibility of initial group differences, underlying trends or correlations between variables that could influence the outcome of a study.
The Role of Age as a Covariate or Factor
Age can be considered both a covariate and a factor in an ANOVA/regression design. As a covariate, age would refer to any variable that is related to the outcome of interest (e.g., IQ in this case) and could be used to explain any differences seen between two groups, such as male and female. Age could also be considered a factor if it is being used as an independent variable in the analysis and its effects on the outcome are being compared between different groups.
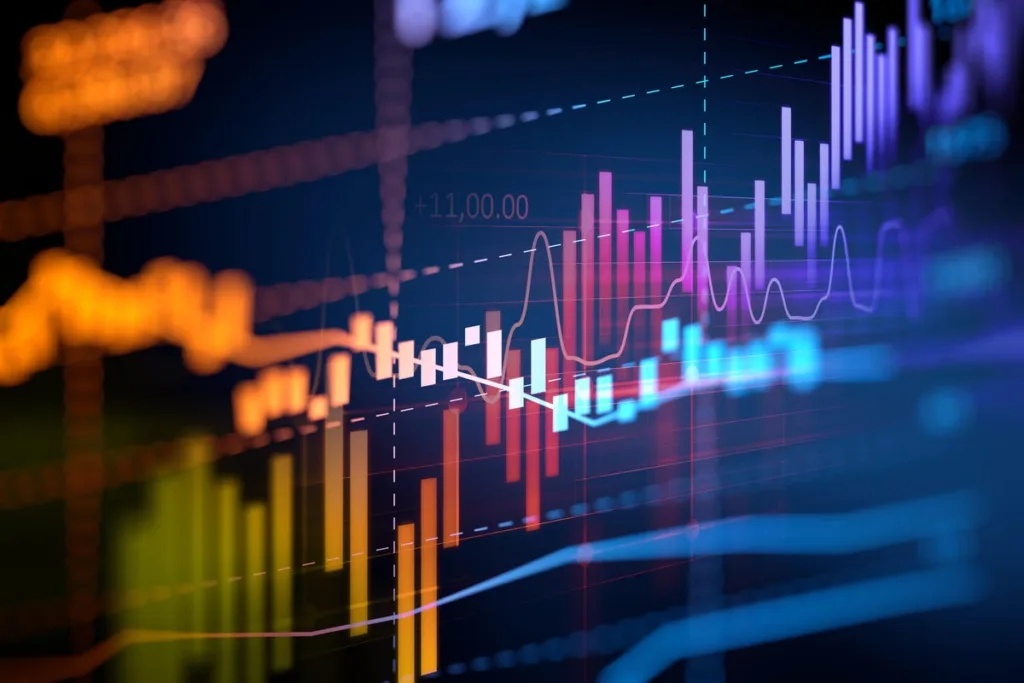
The Role of Gender as a Covariate
Yes, gender is a covariate. A covariate is any variable that is related to the outcome of interest in a study and can be used to control for or explain variation in the results. Gender is a categorical variable that can be used to understand and explain differences between individuals in the results of a study, such as differences in outcomes based on gender. For example, if we were studying reading comprehension, gender could be used as a covariate to investigate whether males or females perform better on reading comprehension tests.
Choosing a Covariate for a Study
When choosing a covariate for a study, it is important to consider the purpose of the study and what information is most likely to be useful in understanding the results. To ensure that the covariates selected are meaningful and relevant to the study, it is important to consider how they are related to the outcome measure. First, one could adjust for covariates that are imbalanced across treatment groups. This would help account for any potential confounding effects due to differences between treatment groups that may not be related to the outcomes of interest. Second, adjusting for covariates correlated with outcome can also help control for potential confounding effects. Finally, adjusting for covariates for which both 1 and 2 hold may be necessary if there is evidence suggesting a possible interaction between them and the outcome measure. Additionally, it is important to assess wether multiple covariates should be included in order to fully capture the relationship between them and the outcome measure.
Can Independent Variables Be Covariates?
Yes, a covariate can be an independent variable. A covariate is a variable that is related to both the outcome and the independent variable of interest. It can affect the relationship between the outcome and the independent variable of interest, so it is important to consider it when analyzing data. An example of an independent variable that could be considered a covariate is age, which could influence the relationship between an outcome (such as disease status) and an exposure (such as smoking). By taking into account age as a covariate, researchers can better understand how smoking affects disease status.
The Use of Covariates in Regression
Covariates are used in regression to account for other factors that may be influencing the outcome of the regression. By including covariates, we can take into account a variety of other factors that could potentially affect our results, such as demographic characteristics, environmental conditions, and lifestyle choices. By accounting for these variables, we can more accurately measure the effect of our independent variable on the response variable. This helps us to better understand how our independent variable is affecting our results and allows us to make more informed decisions based on our analysis.
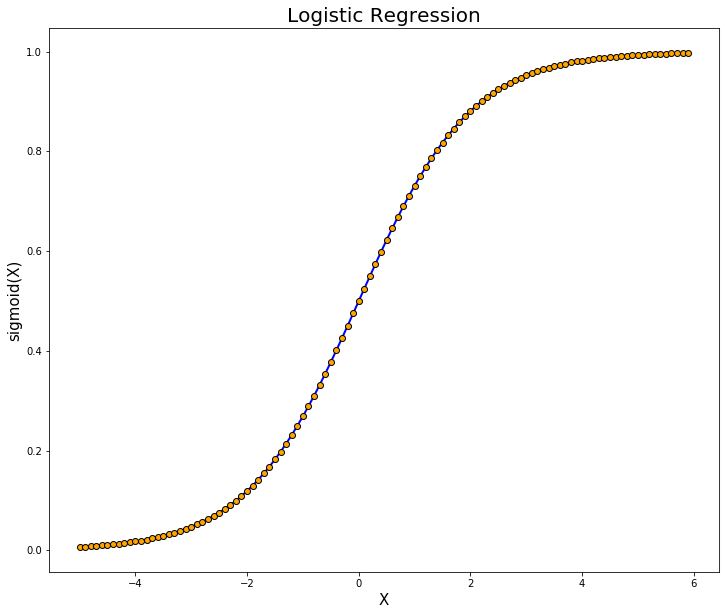
Conclusion
In conclusion, covariates and confounders are both important variables to consider when analyzing the results of a study. Covariates are continuous variables that can explain part of the variability in the outcome, while confounders are variables that may cause spurious associations in research results. It is important to control for both covariates and confounders when interpreting results, as they can skew the data if left unaccounted for. By controlling for these variables, researchers can gain more accurate insights into their study.