Have you ever wondered if the coefficient of determination, also known as R², can be negative? It’s an interesting question that has puzzled many researchers and statisticians. R² is a measure of how well the regression line fits the data points, and it ranges from 0 to 1. A value of 0 indicates that the regression line explains none of the variability in the data, while a value of 1 indicates that the regression line explains all of the variability.
In most cases, R² is positive because the regression line tends to explain at least some of the variability in the data. However, there are situations where R² can be negative. How is that possible, you might ask?
To understand this, let’s first delve into the formula for R². R² is calculated as 1 – (SS_res/SS_tot), where SS_res is the residual sum of squares and SS_tot is the total sum of squares. The residual sum of squares measures the variation that is not explained by the regression line, while the total sum of squares measures the total variation in the data.
When SS_res exceeds SS_tot, the fraction (SS_res/SS_tot) becomes greater than 1, resulting in a negative R² value. This occurs when the regression line is a poor fit for the data and the residual sum of squares is larger than the total sum of squares.
But how can this happen? One possibility is when the intercept or slope of the regression line is constrained. In linear regression, the intercept and slope can be constrained to certain values, which can lead to a poor fit of the line to the data. This constraint can result in a negative R² value.
It’s important to note that with no constraints on the intercept or slope, R² must be positive and equal to the square of the correlation coefficient, denoted as r. The correlation coefficient measures the strength and direction of the linear relationship between two variables. Thus, in the absence of constraints, R² will always be positive.
Can R Squared Can Be Negative?
So, R² is this fancy statistical measure that tells us how well a regression model fits the data. It ranges from 0 to 1, where 0 means the model explains none of the variability in the data, and 1 means it explains all of it. Pretty neat, huh?
Now, here’s where things get interesting. Can R² be negative? Well, technically, yes, it can. But hold on, negative R² is not a good sign. It’s like a red flag waving at us, saying, “Hey, something’s not right here!”
You see, negative R² happens when the residual sum of squares (SS_res) exceeds the total sum of squares (SS_tot). Basically, it means that our model is doing worse than just using the mean value to predict the outcome. Ouch!
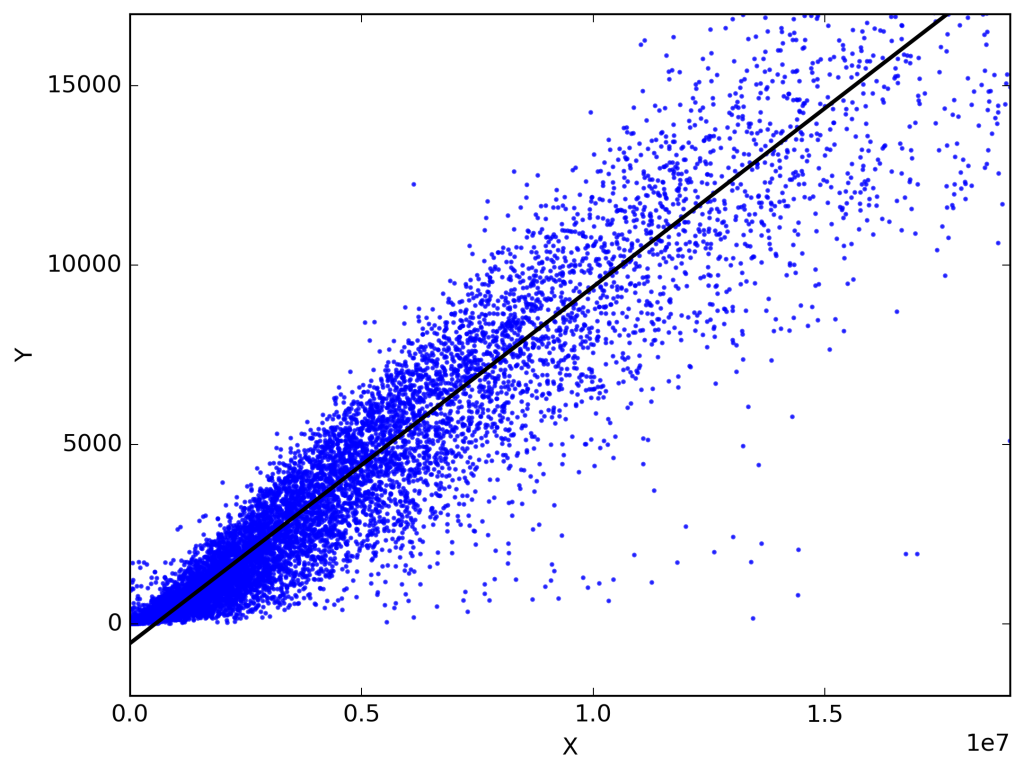
What Does Negative R2 Value Mean?
A negative R2 vlue indicates that there is a negative correlation between the two variables being studied. In other words, as one variable increases, the other variable decreases. The magnitude of the negative R2 value represents the strength of this correlation.
Think of R2 as a measure of how well the data points fit the regression line. A positive R2 value indicates a positive correlation, meaning that as one variable increases, the other variable also increases. A negative R2 value, on the other hand, shows that as one variable increases, the other variable decreases.
To understand this concept better, let’s use a specific example. Suppose we are studying the relationship between temperature and ice cream sales. A negative R2 value would mean that as the temperature increases, the ice cream sales decrease. This suggests that there is an inverse relationship between temperature and ice cream sales – when it gets hotter, people tend to buy less ice cream.
The negative value of R2 can also be thought of as the negative of the slope of the regression line. The slope represents the rate of change between the two variables. In our ice cream example, the negative slope would indicate the rate at which ice cream sales decrease with increasing temperature.
A negative R2 value indicates a negative correlation between the variables being studied. It suggests that as one variable increases, the other variable decreases. The magnitude of the negative R2 value represents the strength of this correlation.
Can R2 Be Positive Or Negative?
R2 can be either positive or negative, depending on the model and the data. R2, also knon as the coefficient of determination, is a statistical measure that represents the proportion of the variance in the dependent variable that is predictable from the independent variables. It ranges from 0 to 1, where 0 indicates that the model does not explain any of the variability in the dependent variable, and 1 indicates that the model perfectly predicts the dependent variable.
When R2 is positive, it means that the model is able to explain some of the variability in the dependent variable. This implies that the independent variables have some influence on the dependent variable, and the model is providing useful information.
On the other hand, R2 can be negative when the model is not able to explain any of the variability in the dependent variable or when it performs worse than a simple average of the dependent variable. However, it’s important to note that negative R2 values are not common in most real-world scenarios and are more often seen in artificial or constrained situations.
In linear regression, for example, where the relationship between the independent and dependent variables is assumed to be linear, R2 can only be negative if the intercept (or perhaps the slope) is constrained. Without any constraints, the R2 must be positive and equal to the square of r, the correlation coefficient.
While it is possible for R2 to be negative under certain circumstances, it is more common for R2 to be positive, indicating that the model explains some of the variability in the dependent variable.
Conclusion
The concept of R² and its potential for being negative is a fascinating and sometimes misunderstood topic. As we have explored, R² is a measure of the strength and direction of the relationship between variables in a regression model. It indicates how well the model fits the observed data, with a value ranging from 0 to 1.
However, it is important to note that negative R² values can only occur under crtain circumstances. Specifically, this can happen when the residual sum of squares (SS_res) exceeds the total sum of squares (SS_tot). In other words, when the model’s errors are larger than the total variation in the data.
This can be the case when there are constraints on the model, such as a fixed intercept or slope. When these constraints are present, the model may not be able to capture the full variation in the data, resulting in a negative R².
It is worth mentioning that in the context of linear regression, where no constraints are imposed, R² is always positive. In fact, it is equal to the square of the correlation coefficient (r), which ranges from -1 to 1. This positive value signifies the strength and directionality of the linear relationship between the variables.
While negative R² values are possible, they are not commonly encountered in linear regression analysis. Understanding the factors that can lead to negative R² values can enhance our interpretation of regression models and help us make more informed conclusions about the relationship between variables.