Residual standard error is a crucial statistical concept that is used to measure the accuracy of a regression model. It is also called the standard deviation of the residuals, which are the differences between the predicted and actual values of the dependent variable.
The residual standard error is calculated as the square root of the sum of squared residuals divided by the degrees of freedom. The degrees of freedom are the number of observations minus the number of parameters in the model. The residual standard error is expressed in the same units as the dependent variable, and it represents the average amount of error in the predictions of the model.
A low residual standard error indicats that the model is a good fit for the data and that the predictions are accurate. A high residual standard error, on the other hand, indicates that the model is not a good fit for the data and that the predictions are not accurate.
The residual standard error is an important measure of the quality of a regression model, and it is often used to compare different models. When comparing models, the model with the lower residual standard error is usually preferred because it is more accurate.
It is important to note that the residual standard error is not the only measure of the quality of a regression model. Other measures, such as R-squared and adjusted R-squared, should also be considered when evaluating the performance of a model.
The residual standard error is a crucial statistical measure that is used to assess the accuracy of a regression model. It represents the average amount of error in the predictions of the model and is used to compare different models. A low residual standard error indicates a good fit for the data and accurate predictions, while a high residual standard error indicates a poor fit and inaccurate predictions.
Is Standard Error The Same As Residual Error?
Standard error and residual error are not the same. Standard error refers to the standard deviation of the sampling distribution of a statistic, such as the mean, and is used to estimate the precision of the sample mean. On the othr hand, residual error, also known as residual standard deviation, is a measure of the variability of the response variable around the fitted regression line. It is calculated as the square root of the sum of squared residuals divided by the degrees of freedom. In other words, residual error represents the amount of variability in the response variable that is not explained by the regression model. Therefore, while both standard error and residual error are measures of variability, they are calculated and used in different contexts and have distinct interpretations.
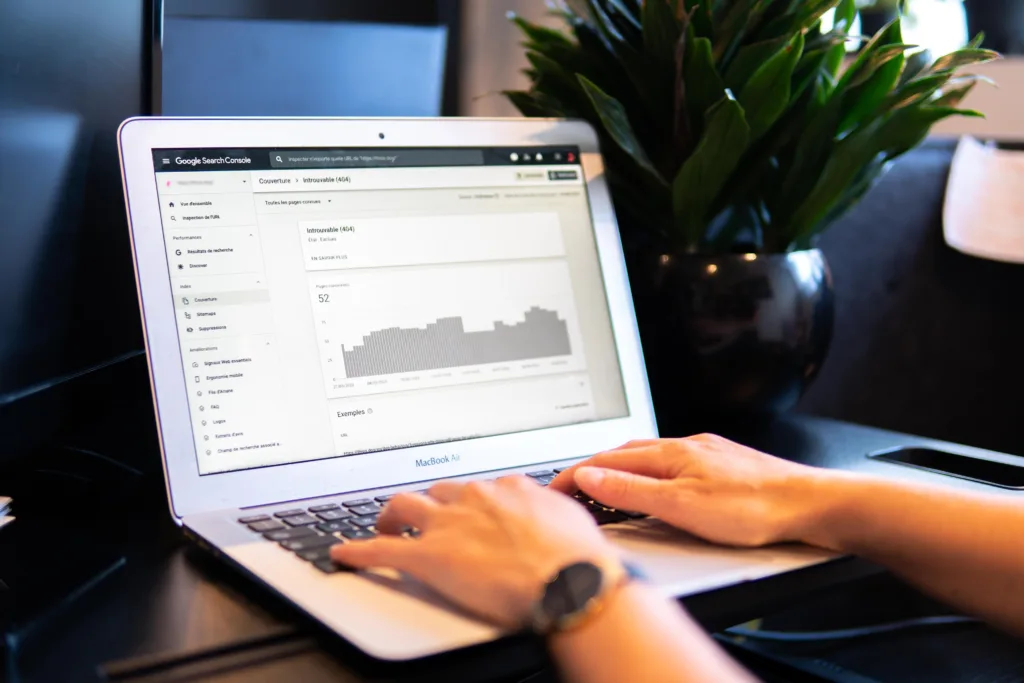
What Is Residual Error Formula?
The residual error formula is a mathematical expression that allows us to calculate the difference between the actual y value and the predicted y value. It is represented by the equation ri=yi−^yi, where ri is the residual error for a specific data point, yi is the actual y value for that data point, and ^yi is the predicted y value for that same data point.
To elaborate, the residual error formula measures how far away the predicted value is from the actual value. If the residual error is positive, it means that the predicted value is lower than the actual value. Conversely, if the residual error is negative, it means that the predicted value is higher than the actual value.
The residual error formula is a crucial tool in evaluating the accuracy of regression models. By analyzing the residual errors of a model, we can identify patterns and trends that may indicae the need for adjustments or improvements to the model. It is important to note, however, that residual errors alone do not provide a complete picture of the accuracy of a model and should be used in conjunction with other metrics and evaluation methods.
What Does Residual Error Show?
Residual error is a statistical term that represents the difference between the predicted value of a dependent variable and its actual observed value. In oher words, it is the deviation of the estimated value from the true value of a quantity of interest. Residual error is used to evaluate the accuracy of a statistical model and to check whether the assumptions of the model are met. A small residual error indicates a good fit between the model and the data, whereas a large residual error indicates that the model may not be appropriate for the data. Residual error is an important concept in many fields, including economics, finance, engineering, and science, where statistical models are used to describe and predict phenomena.
What Is Residual Error In Linear Regression?
In linear regression, residual error refers to the difference between the actual observed value of a dependent variable and the estimated value predicted by the regression model. It represents the amount of variation in the data that cannot be explained by the model and is also known as the “unexplained variability”. Residuals are calculated by subtracting the predicted value from the actual value for each observation in the dataset. These residual values can be positive or negative, depending on whether the actual value is higher or lower than the predicted value. The sum of the squared residuals is used to measure the overall goodness of fit of the regression model. A small residual error indicates a good fit of the model to the data, while a large residual error suggests that the model may need to be revised or improved. It is important to analyze residual plots to check for patterns or trends that indicte the presence of heteroscedasticity or other issues that may affect the validity of the regression model.
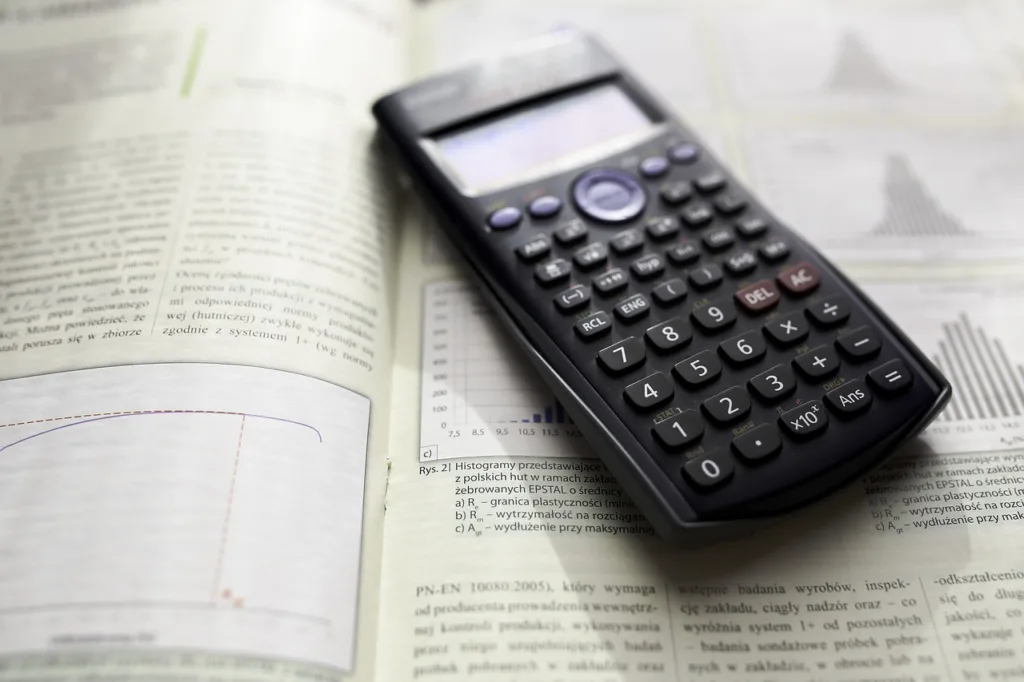
Conclusion
Residual standard error is a vital statistical concept that helps to determine the accuracy of a regression model. It quantifies the variability of the data points around the estimated regression line, and thus, provides a measure of the goodness-of-fit of the model. A small residual standard error indicates that the data points are closely clustered around the regression line, whch suggests a better fit of the model. On the other hand, a large residual standard error indicates that the data points are more dispersed around the regression line, which suggests a poorer fit of the model. Therefore, it is important to calculate and interpret the residual standard error correctly in order to evaluate the reliability and validity of a regression model. residual standard error is a valuable tool for researchers and practitioners who seek to analyze and interpret data accurately and effectively.