The law of iterated expectations is a fundamental concept in probability theory that describes the relationship between conditional expectations of random variables. It is a powerful tool that can be used to calculate the expected value of a random variable by conditioning it on another variable.
The basic idea behind the law of iterated expectations is that the expected value of a random variable can be calculated by taking the average of its conditional expected values over all possible values of another random variable. This can be expressed mathematically as follows:
E(X) = E(E(X|Y))
Where E(X) is the expected value of X, E(X|Y) is the conditional expected value of X given Y, and E(E(X|Y)) is the expected value of E(X|Y) over all possible values of Y.
This formula can be further generalized to include multiple conditional expectations, as follows:
E(X) = E(E(E(X|Y)|Z))
Where E(E(X|Y)|Z) is the conditional expected value of E(X|Y) given Z.
The law of iterated expectations can be applied in a wide range of contexts, including finance, economics, and engineering. For example, in finance, it can be used to calculate the expected return on an investment by conditioning it on varius market factors such as interest rates and inflation.
One important aspect of the law of iterated expectations is that it can be used to derive other important concepts in probability theory, such as the law of total probability and Bayes’ theorem. These concepts are fundamental to many areas of science and engineering, and are essential tools for understanding complex systems and making accurate predictions.
The law of iterated expectations is a powerful concept in probability theory that allows us to calculate the expected value of a random variable by conditioning it on another variable. It has wide-ranging applications in many fields, and is an essential tool for understanding complex systems and making accurate predictions. By using this law, we can gain a deeper understanding of the underlying dynamics of a system and make more informed decisions based on our knowledge of its behavior.
What Is The Adam And Eve Law?
The Adam and Eve law refers to the commandment given by the Creator to Adam and Eve in the Garden of Eden, specifically not to eat from the tree of knowledge of good and evil. This law was a symbol of life, and disobedience was a symbol of death. The consequence of breaking this law was death, which is what Adam and Eve experienced when they disobeyed. The law was meant to be a guide for Adam and Eve to live by, and all they had to do was trust and obey God to live.
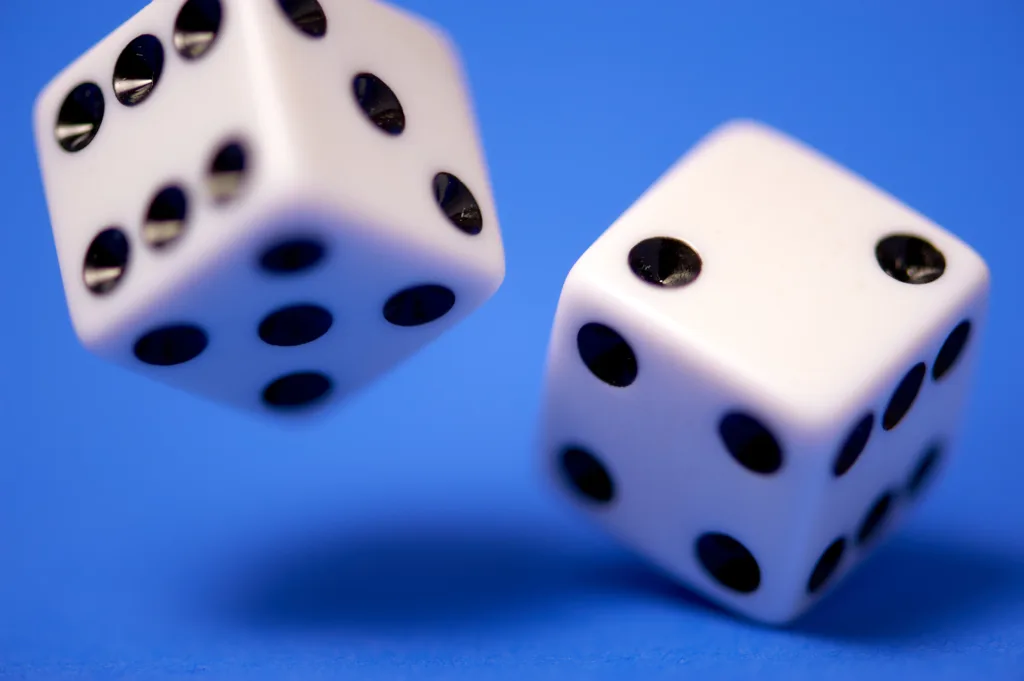
What Is Expectation In Probability Theory?
In probability theory, the concept of expected value plays a crucial role. It is a measure of the central tendency of a random variable, and it represents the average value that one can expect from a large number of independent trials. The expected value is calculated by multiplying the value of each possible outcome by its corresponding probability and adding all the results together.
To illustrate, suppose we have a coin that is fair, meaning that the probability of gtting heads or tails is 0.5 each. If we flip the coin 10 times, we might get 5 heads and 5 tails, or we might get a different outcome. The expected value of the number of heads we get is calculated as follows:
– The probability of getting 0 heads is (0.5)^10 = 0.00098
– The probability of getting 1 head is 10*(0.5)^10 = 0.00977
– The probability of getting 2 heads is 45*(0.5)^10 = 0.04395
– The probability of getting 3 heads is 120*(0.5)^10 = 0.11719
– The probability of getting 4 heads is 210*(0.5)^10 = 0.20508
– The probability of getting 5 heads is 252*(0.5)^10 = 0.24609
– The probability of getting 6 heads is 210*(0.5)^10 = 0.20508
– The probability of getting 7 heads is 120*(0.5)^10 = 0.11719
– The probability of getting 8 heads is 45*(0.5)^10 = 0.04395
– The probability of getting 9 heads is 10*(0.5)^10 = 0.00977
– The probability of getting 10 heads is (0.5)^10 = 0.00098
The expected value of the number of heads is then:
E(X) = 0*0.00098 + 1*0.00977 + 2*0.04395 + 3*0.11719 + 4*0.20508 + 5*0.24609 + 6*0.20508 + 7*0.11719 + 8*0.04395 + 9*0.00977 + 10*0.00098
E(X) = 5
Therefore, we can expect to get 5 heads on average if we flip the coin 10 times.
The expected value is a way to summarize the behavior of a random variable and is calculated as the weighted average of all the possible outcomes, where the weights are given by their corresponding probabilities.
What Is Double Expectation?
Double expectation is a statistical formula that allows you to calculate the expected value of a random variable, X, by conditioning it on anther random variable, Y. The formula can be written as E[X] = E[Y][E[X|Y]], which means that you can find the expected value of X by calculating the expected value of X given each possible value of Y, and then taking the average of those values weighted by the probability of each value of Y occurring.
To apply this formula, you first need to calculate the expected value of X given each possible value of Y. This involves finding the conditional probability distribution of X given Y and then calculating the expected value of X for each value of Y. Once you have these values, you can compute the expected value of X by taking the weighted average of these values with respect to the probability distribution of Y.
Double expectation is a useful tool in statistics and probability theory, as it allows you to calculate the expected value of a random variable under more complex conditions than just the marginal distribution of the variable. It is commonly used in applications such as Bayesian statistics and machine learning, where the relationship between different variables is of interest.
How Do You Find Conditional Expectations?
Conditional expectations can be found using the formula E(X |Y = y) = ∑(x * P(X = x|Y = y)), where X is a random variable and Y is another random variable. In words, this formula calculates the expected value of X given that Y is equal to y.
To find E(X |Y = y), we first need to find the probability distribution of X given that Y = y. This can be done by using the formula P(X = x|Y = y) = P(X = x and Y = y) / P(Y = y), where P(X = x and Y = y) is the joint probability of X and Y taking on the values x and y, respectively, and P(Y = y) is the probability of Y taking on the value y.
Once we have the probability distribution of X given Y = y, we can multiply each possible value of X by its coresponding probability and sum these products to get the expected value of X given Y = y, i.e., E(X |Y = y).
It is important to note that the conditional expectation formula can only be used if the random variables X and Y are dependent. If X and Y are independent, then the expected value of X will not depend on the value of Y, and thus, the conditional expectation formula becomes redundant.
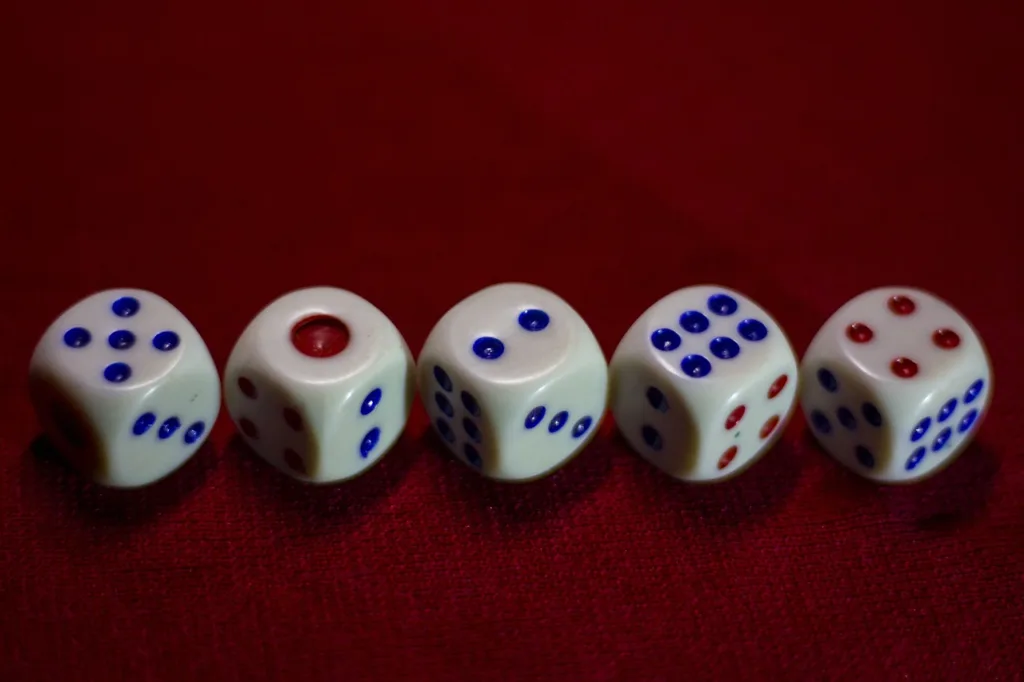
Conclusion
The Law of Iterated Expectations is a powerful tool in probability theory that allows us to calculate the expected vale of a random variable by conditioning it on another random variable. By calculating the expected value of X for each possible value of Y and then taking the average, we can gain valuable insights into the relationship between these variables. This formula is particularly useful when Y has an influence on the value of X, as it allows us to account for this influence and make more accurate predictions. the Law of Iterated Expectations is a fundamental concept in probability theory that is essential for anyone seeking to understand the behavior of random variables and make informed decisions based on this knowledge.