Standard deviation is one of the most widely used measures of variability. It is a statistical tool that measures the amount of variability or dispersion of a set of data points from the mean or average value. It is calculated as the square root of the sum of the squared deviations from the mean divided by the number of data points minus one.
Standard deviation is a useful tool in many fields, including finance, science, engineering, and social sciences. It provides a measure of how much the data is spread out from the mean, which is essential in understanding the distribution of data and making predictions.
However, standard deviation can be affected by outliers. An outlier is an observation that is significantly different from other observations in the data set. Outliers can occur due to various reasons, such as measurement errors, sampling errors, or genuine extreme values.
When outliers are present in a data set, they significantly affect the standard deviation. Outliers tend to increase the standard deviation becase they are far from the mean, making the sum of the squared deviations larger. This can lead to an overestimation of the variability of the data.
To address the issue of outliers, statisticians often use robust measures of dispersion, such as the median absolute deviation (MAD) or interquartile range (IQR). Unlike the standard deviation, robust measures are not sensitive to outliers and provide a better estimate of the variability of the data.
However, in some cases, outliers may be genuine extreme values that reflect the true nature of the data. In such cases, removing outliers may lead to a loss of important information and affect the validity of the analysis. Therefore, it is essential to carefully examine the data and understand the underlying reasons for the outliers before deciding whether to remove them or not.
Standard deviation is a useful tool for measuring variability in data. However, it can be affected by outliers, which can lead to overestimation of variability. To address this issue, statisticians often use robust measures of dispersion, such as the MAD or IQR. However, it is essential to carefully examine the data and understand the underlying reasons for outliers before deciding whether to remove them or not.
How Do Standard Deviations Deal With Outliers?
Standard deviation deals with outliers by allowing for the identification of data points that fall outside a certain range of values. When calculating the standard deviation of a dataset, any values that lie outside a specific number of standard deviations from the mean are considered outliers. These outliers can be removed from the dataset or dealt with in other ways, depending on the specific analysis being performed.
To position the boundaries for identifying outliers, you can specify a multiple of the standard deviation of the outlier field. This multiple can be any positive number, such as 0.5, 1, 1.5, etc. For example, if you specify a multiple of 1.5, the outlier boundaries are 1.5 standard deviations above and below the mean or median of the values in the outlier field.
By usig standard deviation to identify outliers, researchers can ensure that their data analysis is accurate and reliable, as any extreme values that could skew the results are removed or accounted for appropriately.
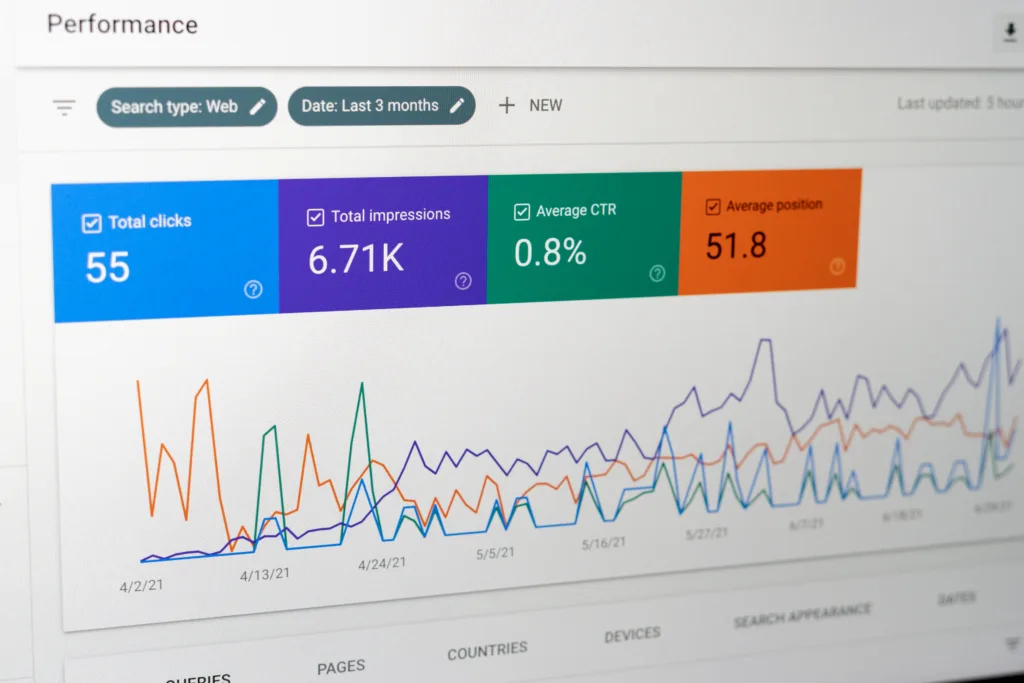
Does Standard Deviation Ignore Outliers?
The standard deviation is a measure of how much the data points in a set vary from the mean. It takes into account all the data points in the set, including outliers. Therefore, it does not ignore outliers. In fact, outliers can have a significant impact on the standard deviation, especially if they are far from the mean. The standard deviation is calculated by taking the square root of the sum of the squared differences between each data point and the mean, divided by the number of data points minus one. This means that outliers, which are farther from the mean than most other data points, will contribute more to the overall variance of the data set and therefore have a larger impact on the standard deviation. However, if an outlier is removed from the data set, the mean will change and so will the standard deviation. If the outlier was a larger vale than the mean, the standard deviation will decrease, while if it was a smaller value than the mean, the standard deviation will increase.
When You Remove Outliers What Happens To The Standard Deviation?
When outliers are removed from a data set, it is expected that the standard deviation will decrease. This is because outliers have a significant impact on the calculation of standard deviation, which is a measure of the spread of data from the mean. If the data set contains outliers, the deviation from the mean will increase, resulting in a higher standard deviation.
By removing outliers, the calculation of standard deviation will be based on the values that are closer to the mean, which is likely to be a btter representation of the population characteristics. Therefore, the standard deviation will be lower, indicating that the data is less spread out and more consistent.
It is important to note that the impact of outliers on standard deviation depends on the number and magnitude of outliers present in the data set. In some cases, the removal of outliers may not result in a significant change in the standard deviation. However, it is generally advisable to identify and remove outliers to obtain a more accurate representation of the data.
Removing outliers from a data set is likely to result in a decrease in the standard deviation, as the calculation of standard deviation is based on the values that are closer to the mean.
Do Outliers Inflate Standard Deviation?
Outliers can inflate the estimate of the standard deviation. Standard deviation is a measure of how spread out the data is aound the mean. Outliers are data points that are significantly different from the rest of the data. When calculating the standard deviation, outliers can skew the estimate by making it appear as if the data is more spread out than it actually is. This is because the standard deviation is calculated by taking the square root of the sum of squared differences between each data point and the mean. Outliers with large differences from the mean will have a greater effect on the sum of squared differences and therefore increase the estimate of the standard deviation. To avoid this issue, it is important to identify and remove outliers before calculating the standard deviation.
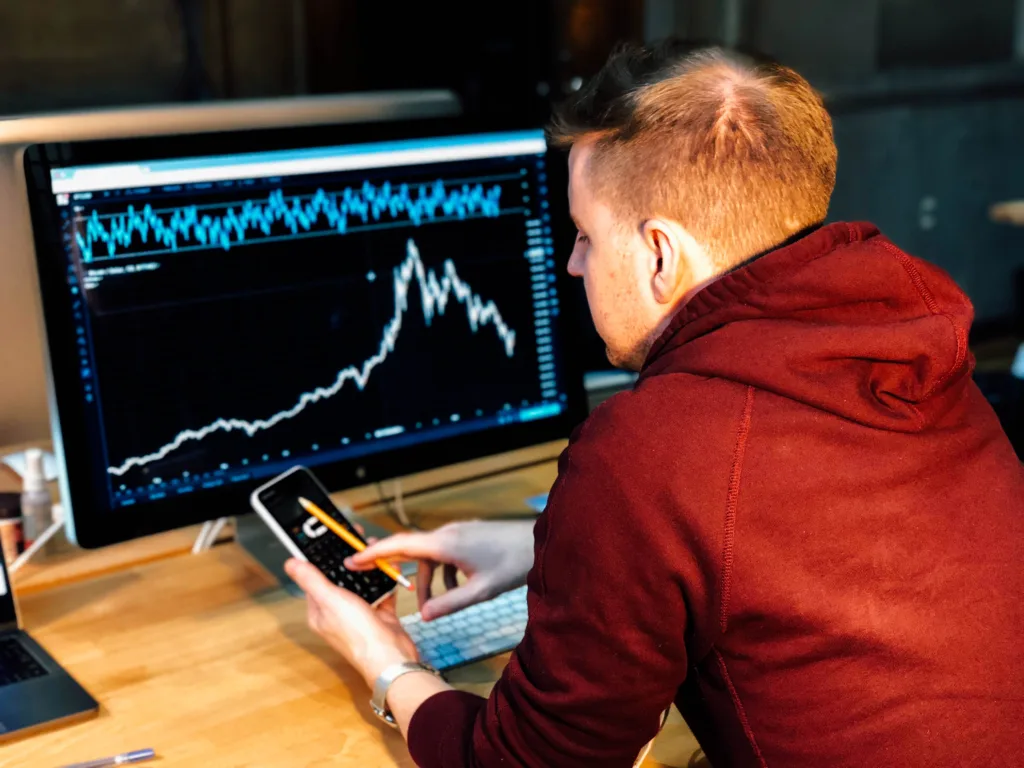
Conclusion
The standard deviation is a valuable statistical tool that measures the spread of data around the mean or median. It is prticularly useful in identifying outliers or extreme values in a data set. By specifying a multiple of the standard deviation, we can position boundaries to identify outliers and determine their impact on the data set. Removing outliers can lower the standard deviation, providing a more accurate representation of the population characteristics. The standard deviation is an essential tool in many fields, including finance, engineering, and science, as it helps to make informed decisions based on data. Understanding the concept of standard deviation is crucial to analyze data accurately and make informed decisions based on the results.